Abstract
彩票大全软件:Background
Non-invasive prenatal testing has the potential to be a useful genetic screening tool in Australia. However, concerns have been raised about its cost, commercial provision, the psychological impacts of the screening process, and disparities in access experienced by rural and regional communities.
彩票大全软件:Aims
The aims of this study are (1) to estimate Australian preferences for features of prenatal screening; (2) to explore potential variations in preferences between metropolitan and rural/regional communities; (3) to estimate the extent to which respondents are willing to trade-off between attributes, using willingness to pay (WTP) and willingness to wait estimates.
彩票大全软件:Methods
A discrete choice experiment (DCE) was conducted with 12 choice tasks. The DCE recruited participants from metropolitan (n?=?160) and rural/regional (n?=?168) locations across Australia. Mixed logit and latent class analyses were conducted and WTP and willingness to wait were calculated.
彩票大全软件:Results
Both metropolitan and rural/regional preferences were significantly impacted by the false-positive rate, false-negative rate, and cost. In addition, rural preferences were significantly impacted by the scope of the conditions covered, the inconclusive rate, and wait times. The number of screening tests and revealing the sex of the foetus were not significant within either group. Willingness to pay estimates ranged from AU$13 to avoid a test with a 1% increase in the false-positive rate to AU$323 to screen for a wide range of conditions.
彩票大全软件:Conclusions
This study highlights the importance of considering differing preferences between rural and metropolitan populations when delivering prenatal screening. Further, this study provides Australian-specific WTP estimates to be incorporated into economic evaluations.
彩票大全软件:Similar content being viewed by others
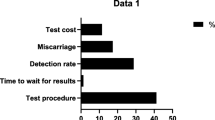
彩票大全软件: Eliciting women’s preference for prenatal testing in China: a discrete choice experiment
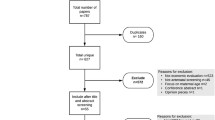
彩票大全软件: The role of information provision in economic evaluations of non-invasive prenatal testing: a systematic review
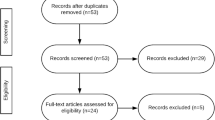
彩票大全软件: Preferences for aspects of antenatal and newborn screening: a systematic review
彩票大全软件:Explore related subjects
Discover the latest articles and news from researchers in related subjects, suggested using machine learning.Avoid common mistakes on your manuscript.
Non-invasive prenatal testing (NIPT) could be a valuable genetic screening tool in Australia. However, concerns exist about its cost, commercial provision, psychological impacts, and access disparities in rural areas. |
This study found that while metropolitan preferences were shaped by the accuracy and cost of screening tests, rural preferences were influenced by a broader range of screening features. |
This study provides Australian-specific WTP estimates to be incorporated into economic evaluations, which could directly inform future decisions around NIPT that explicitly account for Australian preferences. |
1 Introduction
Recent advancements in technology have led to the introduction of non-invasive prenatal testing (NIPT). Non-invasive prenatal testing uses cell-free DNA (also referred to as cell-free DNA screening) [1], circulating in maternal plasma to detect chromosomal abnormalities in the foetus [2]. Non-invasive prenatal testing offers a higher detection rate and lower false-positive rate for the three common trisomies (T21, Down syndrome; T18, Edwards syndrome; T13, Patau syndrome) compared to traditional combined first-trimester screening (cFTS), which involves an ultrasound and maternal serum biochemical marker evaluation of beta human gonadotropin and pregnancy associated plasma protein A. Non-invasive prenatal testing offers additional advantages, such as the capability of detecting a broader range of conditions, including microdeletions and duplications and sex chromosome conditions (e.g., Turner syndrome and Klinefelter syndrome), as well as revealing the sex of the foetus [3, 4]. However, NIPT is associated with a higher cost compared to cFTS, displays decreased accuracy when applied to the broader range of conditions, and these conditions present with variable phenotypes. There are two approaches for using NIPT: a first-line test offered to all pregnant women; or a second-line test offered only if there is a high-risk result on cFTS. In Australia, cFTS receives a public subsidy, whereas NIPT predominantly?does not. Non-invasive prenatal testing is gaining popularity, and this is raising equity concerns given the lack of public funding and absence of a formal implementation plan [5, 6].
Applications to the Australian government seeking public subsidy for NIPT have been unsuccessful. Although the Australian government accepted that NIPT (for trisomies 21, 18 and 13) was clinically efficacious and superior to cFTS, it was not considered to be cost effective compared to cFTS (at an estimated AU$510,769 per extra trisomy detected [7]. The consideration highlighted the challenges in assessing the economic impact of including NIPT on the Medicare Benefits Schedule (MBS; a listing of the health services subsidised by the Australian Government). These challenges included accounting for the psychological consequences of the screening process and addressing disparities in rural and regional areas with limited access to ultrasounds (an essential component of cFTS) and follow-up invasive testing. Indeed, the government advisory committee noted that a geographically restricted MBS item, targeting rural and regional areas, might be an approach for future consideration. Recent guidelines from the Royal Australian and New Zealand College of Obstetricians and Gynaecologists (RANZCOG) advocate for research to explore preferences for prenatal screening, particularly in rural and regional areas [8]. This research will assist in developing a formal and equitable implementation model for prenatal screening in Australia.
Much has been written about preferences for prenatal screening overseas. A recent systematic review identified 46 quantitative and mixed-method studies and highlighted the complex decision-making processes involved in prenatal screening. Decision-making factors extended far beyond the diagnostic rate, to include procedural aspects (e.g., information provision, convenience and wait time for results) and the psychological impacts of receiving false or uncertain results, which can be exacerbated by longer wait times. Despite the large number of studies identified, none were conducted in Australia, where preferences have been shown to differ in the context of prenatal diagnostic testing [9]. Preferences of the Australian public for cFTS have been assessed prior to the introduction of NIPT [10], and there have been studies on the preferences of pregnant women undergoing NIPT [11, 12], as well as a recent investigation into preferences specific to Western Australia (WA) [13]. However, there remains a gap in the evidence around Australian-wide preferences for prenatal screening in a context where NIPT is locally available.
To explore Australian preferences for prenatal screening, we have used a discrete choice experiment (DCE). Discrete choice experiments are stated preference methods used to understand the trade-offs people make between programme features (known as attributes) [14]. This study aims to (1) explore Australian preferences for features of prenatal screening; (2) explore potential variations in preferences between metropolitan and rural/regional communities; (3) estimate the extent to which respondents were willing to trade-off between attributes, including willingness to pay (WTP) estimates, which could be used in future economic evaluations.
2 Methods
彩票大全软件:2.1 Study Design
Preferences for prenatal screening were elicited using a DCE, designed and developed according to the International Society for Pharmacoeconomics and Outcomes Research (ISPOR) Conjoint Analysis Task Force for good research practices checklist [15]. This DCE presented participants with a series of questions, asking them to choose between three scenarios: two unlabelled alternative approaches to prenatal screening, or ‘no screening’. Each alternative included attributes (i.e., features of the programme such as the cost and false-positive rate) with varying levels. By administering multiple choice tasks to each participant, we were able to quantify the relative importance of attributes and their levels. By including cost as one of the attributes, we were able to calculate the WTP for particular attributes of the programme. Calculating WTP provides an avenue to integrate intermediate (i.e., the impacts of receiving false or uncertain results) and process outcomes into economic evaluations and applications for public funding [16].
彩票大全软件:2.2 Setting and Participants
The DCE was conducted as a web-based survey through Qualtrics. Discrete choice experiment participants included: (A) adults (aged 18 to 45 years) based in Australia, who have been pregnant or are potentially interested in becoming pregnant, or (B) partners of adults who would meet the definition of group A, whose actual partner may or may not be a participant in the study. People who could not speak English were excluded from the study. The DCE population will be referred to as the ‘general public with an interest in pregnancy’.
This population was chosen was to ensure the survey was presented only to those for whom it is relevant and enhances the relevance of the DCE choice tasks for respondents. In contrast to the majority of previous studies which focus on women [17], all genders were included in this study to recognise the role partners play in the decision-making processes [18, 19].
Participants were recruited through an independent external web-panel provider (Dynata). Participants were recruited from the panel provider’s existing online research panel and were emailed a link to the survey from the panel provider. Quota sampling was used to ensure diversity across gender and geographical location, ensuring we had at least 50% of the sample recruited from non-metropolitan (i.e., inner regional, outer regional or remote) areas across Australia. Data were collected between February 15th and April 5th, 2024. The study was approved by the University of Sydney Human Research Ethics Committee [2023/170].
彩票大全软件:2.3 Attributes and Levels
The DCE attributes and levels were informed by a review of the literature, consultation with an expert advisory group (EAG) and a series of focus group discussions. A rapid literature review was performed to understand the broad scope of attributes that might impact on preferences for prenatal screening. Details and results can be found within the Supplementary materials. Consultation with the EAG was used to identify priority attributes for discussion within the focus groups. The EAG included a diverse group of relevant healthcare professionals currently working in Australia, including four obstetricians, a genetic counsellor and a midwife, as well as an ethics researcher specialising in prenatal screening, and a person with lived experience of NIPT who received a false-positive result. The priority attributes chosen for focus group discussion can be found within the Supplementary materials.
Three focus groups were set up in New South Wales, Australia. The focus groups comprised 25 participants of childbearing age from the general public with an interest in pregnancy. Focus groups took place face-to-face in metropolitan and rural/regional areas, and online via videoconference recruiting from rural/regional areas. Discussions were transcribed and analysed thematically using NVIVO qualitative software. An extensive description of the focus group methodology is provided elsewhere [20] . Focus group results were discussed with the EAG to determine the final attributes and levels for inclusion within the DCE. The eight attributes included within the DCE can be found in Table 1. The EAG provided valuable input on the wording of attributes and levels to ensure participant understanding, e.g., ‘chance of a missed case’ and ‘chance of a false alarm’ representing false negatives and false positives. Attributes which involved risk estimates were presented using two formats: numerical (expressed as the number of babies or tests out of 100) and visual (using an icon array, consisting of a matrix of 10×10 squares with colours representing proportions).
The selected levels were based on the literature, focus group discussions, and consultations with the EAG. The levels for wait times of the screening process were developed based on feedback from focus groups and are intended to incorporate the wait times experienced by individuals in rural and regional areas, as well as those receiving complicated or uncertain results. The levels for the false-positive rate reflect the accuracy of NIPT in detecting the three common trisomies (0.1%) [2] and recent evidence that the false-positive rate for rare autosomal trisomies can be as high as 55% [21]. The cost levels were based on the current price of NIPT (for three common trisomies) at AU$450 in Australia [5]. We included extreme values of AU$0, representing an ‘ideal’ and hypothetical publicly funded system, and AU$1000, reflecting a hypothetical commercial price for expanded NIPT (i.e., NIPT which tests for the broader range of conditions). All levels were reviewed by the EAG and were considered acceptable in light of the current evidence. The structure of the survey and information presented to participants prior to completing the choice tasks can be found within the Supplementary materials.
彩票大全软件:2.4 Cognitive Interviews
The DCE survey was tested through cognitive (think aloud) interviews to assess understanding and comprehension of the survey, using a convenience sample (n?=?10). A number of changes were made based on the feedback from the cognitive interviews including formatting changes (e.g., creating space between the columns which represent the screening approaches; presenting the scenario in dot points) and changes to the wording of the scenario, question, and attribute levels to improve clarity (e.g., adding clarification that the focus of the survey is the screening test and not the diagnostic test). An example of the final choice task can be found in Fig. 1.
彩票大全软件:2.5 Experimental Design
An online pilot survey was used to obtain priors on the first 35 respondents recruited through the web-panel provider. Ngene version 1.4.0 was used to generate a D-efficient design with 60 choice tasks in five blocks of 12 choice tasks. The DCE was carried out in two steps. In the first step, participants were presented with two alternatives and an opt-out option. The opt-out option aims to reflect a real-life situation where they can decide not to partake in the screening programme. If a participant decided to opt out, they were then presented with an additional question forcing them to choose between the two alternatives. The forced choice was included in case the opt-out option caused a significant decrease in the data available to analyse preferences [15]. A sample size of 328 participants was used for the full DCE. Although sample size calculations are not required for DCEs [22], the chosen sample size exceeds the minimum sample size using the rule of thumb method proposed by Orme, which accounts for two-way interactions (n?=?222) [23]. See the Supplementary material for more information on survey design and sample size.
彩票大全软件:2.6 Analysis
Microsoft Excel was used to generate descriptive statistics and NLOGIT version 6 was used for the DCE analyses. Based on exploration of the pilot data, it was decided that participants who completed the survey in less than three minutes would be excluded from the main analysis. These respondents were considered to be ‘potential bots’, given that it was estimated to take 2–3 minutes to navigate the survey by clicking 'Next' as quickly as possible. To further minimise the risk of bots, respondents were excluded if they had a ReCAPTCHA score below 0.5 and failed the white text 'bot' question, which is written in the same colour as the background and cannot be seen by a person.
The initial analysis employed a multinomial logit model (MNL) as a baseline for developing more sophisticated models. Within the MNL model, all attributes were tested as categorical (effects coded) and continuous variables to establish the best model fit. The final model included variables Conditions covered and Sex reveal as categorical variables using effects coding, and all other attributes were coded as continuous variables. Although DCEs are commonly analysed using an MNL model, it does not allow preference heterogeneity to be assessed [24]. To overcome this limitation, the data were analysed using a mixed-logit panel model and latent class panel model.
A mixed logit model yields both a mean and standard deviation effect across the sample, reflecting the differences in preferences among respondents. The mixed logit model specified cost to have random parameters with a truncated triangular distribution, as a priori cost is known to have a negative coefficient. All other attributes were coded to have normally distributed random parameters. Dummy variables were created to distinguish between metropolitan and rural (remote and regional) respondents. These dummy variables were interacted with all attributes to assess how geographical location influenced preferences. Other sociodemographic characteristics (age, education, income, termination attitudes and gender) were effects coded and explored as main effects. The mixed logit analysis was conducted using 10,000 Halton draws. The final mixed logit model results are presented as the estimated β parameters, p-values (with the statistical significance threshold of p < 0.05) and the standard deviations (SD) and p-values and confidence intervals of the random parameters. The utility function and results for alternative model specifications are provided in the Supplementary materials.
Latent class (LC) models with a different number of classes (one to eight) were also estimated to determine the ideal number of classes to fit the data [24]. The LC model with the best fit included four classes. For all models, model fit was compared using the Akaike information criterion (AIC), pseudo R2 and Log likelihood of model (LL) [15].
Marginal rates of substitution (trade-offs) were estimated for significant attributes (p < 0.05) as benefit/harm trade-offs and WTP estimates. These estimates were calculated using the results from the mixed logit model and calculated separately for metropolitan and rural groups (based on interactions between attributes and geographic group). Benefit/harm trade-offs were calculated for significant attributes in relation to wait time (weeks). This involved calculating the ratio of an attribute’s parameter estimate (or difference between level estimates, if categorical) to the negative of the parameter estimate of wait time. The WTP estimates represent how much participants are willing to pay (in AU$) for specifc features of screening. This involved calculating the ratio of an attribute’s parameter estimate (or difference between level estimates, if categorical) to the negative of the parameter estimate of cost [25]. An additional analysis was conducted to estimate the WTP to avoid an increase in the false-positive rate from 0.1% to 55%. This estimate is based on evidence regarding the false-positive rates associated with screening for three common trisomies [2] and a wide range of other conditions [21]. This analysis assumed a linear WTP and multiplied the WTP estimate for a unit change in the false-positive rate by 54.9 (the difference between 55% and 0.1%). A supplementary analysis was performed including ‘potential bots’, using a mixed logit model.
3 Results
彩票大全软件:3.1 Participants
In total, there were 374 eligible respondents, based on the inclusion criteria. Forty-six respondents were then excluded from the analysis, including 34 respondents who completed the survey in less than three minutes (potential bots), and 12 others who failed the additional quality control measures, including low ReCAPTCHA scores and responding to a white bot question. There were 328 respondents included within the main analysis, and the mean time to complete the survey was 17.6 minutes, falling within the expected range.
The sociodemographic characteristics of included respondents are reported in Table 2. There was a relatively even spread across gender, age ranges, and education status of respondents. The majority of respondents had no experience with prenatal screening or were unsure (63%). The sample consisted of 160 people (49%) living in a major city, 103 (31%) in inner regional locations, 61 in outer regional, remote or very remote locations (19%) and four respondents were unsure (1%). Metropolitan and rural (including inner regional, outer regional, remote and very remote locations) respondents were similar across the majority of sociodemographic characteristics. However, some differences were seen in age distributions and sex across metropolitan and rural respondents.
彩票大全软件:3.2 Mixed Logit Model
The mixed logit model had a log likelihood function of ?2669, AIC/N of 1.581 and R2 of 0.292. The analysis is based on the three alternatives. Since we collected sufficient data, the forced choice was not analysed. The estimated coefficient for significant attributes (p < 0.05) had the expected sign providing support for the validity of the model. Results for the mixed logit model are discussed below and presented in Table 3. The supplementary analysis including ‘potential bots’ is presented in the Supplementary materials; the inclusion of these respondents did not have a substantial impact on the results.
3.2.1 Preferences
Respondents strongly favoured prenatal screening over no screening (β?=???3581, p-value 0.000). Metropolitan respondents preferred a screening test with a lower false-positive rate, lower false-negative rate and lower cost. The conditions covered, inconclusive rate, sex reveal, number of tests and wait time were not found to be significant within the metropolitan group, and the confidence intervals ranged from negative to positive for each of these attributes. Within the rural group, a greater number of attributes were found to impact on preferences, with respondents preferring a test which covers a broader range of conditions, lower false-positive rate, lower false-negative rate, lower inconclusive rate, shorter wait time and lower cost. The number of tests and sex reveal were not found to be significant. A large number of attributes were found to have statistically significant and relatively large standard deviations, indicating preference heterogeneity. Although including sociodemographic characteristics as main effects improved the model fit, these factors were not statistically significant predictors.
彩票大全软件:3.3 Marginal Rates of Substitution
3.3.1 Benefit to Harm Trade Offs
Metropolitan respondents were willing to wait the longest to avoid an increase in the test cost of $100 (at 4?weeks), and the least amount of time to avoid a test with a 1% increase in the false-positive rate (at 0.6?weeks). Rural respondents were willing to wait the longest to screen for a wide range of conditions (instead of only the three trisomies—at 10.3 weeks), and the least amount of time to avoid a test with a 1% increase in the false-positive rate (at 0.5?weeks). The results can be found in Table 4.
3.3.2 Willingness to Pay
For metropolitan respondents, WTP was highest to avoid a 1% increase in the false-negative rate (at AU$30), and lowest to avoid a 1% increase in the false-positive rate (at AU$13). For rural respondents, WTP was highest to screen for a wide range of conditions (instead of only the three trisomies—at AU$323), and lowest to avoid a 1% increase in the false-positive rate (at AU$16). The results can be found in Table 5.
Assuming that WTP is linear (where respondents are willing to pay AU$16 for each 1% increase in the false-positive rate), respondents would be willing to pay AU$878 to avoid an increase in the false-positive rate from 0.1% to 55%.
彩票大全软件:3.4 Latent Class Model
The results were also analysed using latent class (LC) analysis. However, the LC model did not offer an improvement over the mixed logit models, and no significant predictors of class membership emerged. Therefore, the results for the LC model have not been discussed and are instead provided in the Supplementary materials.
4 Discussion
This DCE investigated Australian preferences for prenatal screening in both rural and metropolitan locations across the country. The findings revealed that Australians have a strong preference for undergoing prenatal screening. Metropolitan respondents’ preferences were significantly influenced by the accuracy and cost of the screening test. In contrast, preferences of rural respondents were influenced by a broader range of factors including the scope of conditions covered, test accuracy, wait times and cost. The number of screening tests and revealing the biological sex of the foetus were not significant features within either group. In scenarios with high levels of false-positive rates, consistent with expanded NIPT, rural respondents prioritised the false-positive rate over the scope of conditions covered by the screening test. Rural and metropolitan respondents displayed similar preferences for attributes significant to both groups, where benefit to harm trade-offs and willingness to pay results were comparable.
Rural preferences for prenatal screening were influenced by a broader range of prenatal screening features compared to the metropolitan group. Rural respondents preferred a test which screened for a wide range of conditions, a lower inconclusive rate and shorter wait times. In contrast, metropolitan preferences were not found to be significantly influenced by these features of screening. It was anticipated that rural respondents would have a greater number of concerns given the additional challenges encountered during pregnancy by individuals in rural Australia, including difficulties accessing prenatal screening and follow-up services due to location and socioeconomic factors [7, 26,27,28,29]. Additionally, there are lower levels of support and resources available to raise a child with a disability, increasing the implications of screening [29]. As expected, wait times significantly impacted the preferences of rural respondents. This is likely due to rural communities being familiar with the effects of wait times [30], whereas for metropolitan participants, 10 weeks may not seem like a realistic scenario. A surprising result was the similarity in the influence of cost between both groups, along with comparable WTP estimates for different features of screening. We expected cost to be a greater concern to rural respondents given they generally have a lower socioeconomic status, and the cost of prenatal screening and follow-up services present greater risk to their financial protection. The similarity in results could be due to the similar income distribution across respondent groups, and/or due to the indirect costs of screening (e.g., travel, accommodation and resulting loss of income) not being considered within the DCE. These findings are consistent with the conclusion that the lower uptake of NIPT in rural Victoria is likely driven by socioeconomic factors, rather than valuing the features of screening less than metropolitan communities [31]. Further research is needed to estimate current uptake of NIPT in rural areas across Australia and to understand whether publicly funded NIPT could help reduce inequities. These findings are specific to the Australian context; however, they may be relevant for other Western countries which do not publicly fund NIPT.
Our results suggest a complex interplay between preferences for the scope of the test and the false-positive rate. Interestingly, metropolitan preferences were not significantly influenced by conditions covered, unlike within the rural group. The qualitative literature describes two models of thought regarding testing for a broader range of conditions. The first model is underpinned by the desire “to have a fuller understanding of the health of their baby and their pregnancy” and resolve uncertainty [6]. While the second model revolves around not all information being beneficial, with increased information leading to results that are unanticipated and not reassuring [6, 12]. These divided views are also present when considering genomic screening in a broader context [32]. Our findings suggest the rural group aligns more with the first model, valuing comprehensive information to resolve uncertainties. This preference may be influenced by the limited support available for raising a child with a disability in rural areas. Meanwhile, the metropolitan group shows more variability in their decision making, possibly reflecting the broader range of experiences and resources available in urban settings. However, rural respondents only prioritised the scope of conditions covered when false positives were low. In reality, low false-positive rates are consistent with detecting only the three common trisomies [2]. When considering a broad range of conditions, the false-positive rate can be as high as 55% [21]. At this extreme value, rural respondents prioritised the false-positive rate over the scope of conditions covered.
Within the Australian context, clinicians have raised concerns about expanding the scope of NIPT. This is due to the potentially high levels of false-positive rates, variable phenotypes and lack of resources in the Australian health system to appropriately deliver and manage such results [6, 33]. In WA, preferences for an increased scope of testing (100–400 genetic disorders compared to three common trisomies and sex chromosome conditions) were found to be of similar magnitude to the preference for high accuracy (positive predictive value) [13]. Internationally, studies have shown varying priorities amongst women. A DCE conducted in the Netherlands found conditions covered to be the most important attribute; however, only low levels of false-positive rates were considered [34]. In contrast, two international DCEs found respondents prioritised a relatively small reduction in accuracy over the scope of the test [35, 36]. An international survey found that across multiple countries (including Australia), all countries except China preferred a broad rather than a targeted test, although this was not traded off against accuracy [9]. Our findings demonstrate that the Australian public preferences align with clinicians’ concerns when they are presented with high false-positive rates, emphasising the importance of communicating the accuracy of the prenatal screening test.
This study quantifies the impact of intermediate and process outcomes on preferences through WTP estimates. These estimates ranged from AU$13 to avoid a one percent increase in the false-positive rate to AU$51 to avoid a one percent increase in the inconclusive rate. These findings align with the qualitative literature, which highlight the stress and anxiety associated with wait times and the uncertainty regarding false or inconclusive results [37]. Qualitative studies have reported that anxiety around wait times stem from the concern that pregnant individuals might be forced to make rapid healthcare decisions that affect them, their children and their family [38]. In line with our results, a recent DCE conducted in WA found women were willing to pay between AU$281 and AU$1256 to avoid a 49% decrease in accuracy, noting they were looking at a different application of testing (even larger scope including single gene disorders) [13]. A DCE conducted in the Netherlands reported similar, although somewhat larger, WTP estimates compared to our results, with women willing to pay 23 euros (~AU$37) for results to be received one week earlier and 112 euros (~AU$182) for a percentage point lower false-positive rate [34]. A DCE conducted in Singapore calculated even larger WTP estimates [39]. The importance of process and intermediate outcomes is also supported within the broader literature of genetic and genomic testing, with three DCEs finding that Australians are willing to pay AU$2340 to be very likely to improve the process of medical care (compared to not likely) in the context in paediatric genomic sequencing, AU$1625 in the context of symptomatic adult genomic sequencing and AU$1177 in the context of at-risk adult genomic sequencing [40]. These findings collectively highlight the importance of considering both intermediate and process outcomes in policy decisions.
The WTP estimates presented in this study offer a pathway to directly integrate the impacts of process and intermediate outcomes into economic evaluations. Considering the current estimated cost of NIPT is AU$450 [5], it is likely that including these estimates into economic evaluations will influence the cost effectiveness of NIPT. Various options have been proposed in the literature. The more conventional approach is to incorporate WTP estimates through a cost-benefit analysis [16]. A less conventional approach would be to include the WTP estimates within the cost side of a cost-utility analysis [41]. Among economic evaluations of NIPT, it is rare to consider the associated intermediate and process outcomes [42]. Evaluations which have attempted to include these outcomes have relied on a time trade-off study, which found only minor decrements in utility [43]. This contrasts with qualitative literature that suggests these outcomes have considerable impact. The study authors hypothesise that the discrepancies may be due to the time trade-off methodology used, which involved multiple complex scenarios and a trade-off with death. The challenge of including process and intermediate outcomes into economic evaluations is not limited to prenatal screening but is present more broadly in genomic testing [44]. How to include evidence from stated preference research in health technology assessments is also the subject of current debate [16, 45,46,47]. Further research is needed to explore the implications of including WTP estimates for process and intermediate outcomes within economic evaluations, and the resulting impacts on decision making.
5 Limitations
A number of limitations should be considered when interpreting the results of this study, including the population, recruitment methods and sample size. This study includes members of the general public who have an interest in pregnancy. Whilst this means respondents were more likely to be engaged in the content of the task, it also means that the WTP estimates may not be representative of the general population. This has implications for public funding decisions, given that Australian guidelines currently recommend using public preferences rather than patient preferences, noting our sample is broader than the patient population [48]. We would expect the WTP estimates to be lower if individuals with no interest in pregnancy were included. However, these estimates are consistent, although somewhat lower, than a DCE conducted in WA, which includes all women aged between 18 and 45?years [13]. Although steps were taken to avoid bots, including trap questions, ReCAPTCHA scores and removing respondents who took less than 3 minutes to complete the survey, there remains a possibility of their occurrence. The focus groups were conducted in one state (New South Wales), while the DCE was conducted across Australia. The sample size was substantial for the primary analysis; however, the sample size limited our ability to analyse preferences for different subgroups (e.g., different income levels, age, termination attitudes), which is likely to explain why the latent class model did not offer improvements and why the sociodemographic predictors in the mixed logit model were not significant. Lastly, this survey required relatively high levels of literacy and excluded people who could not speak English.
6 Conclusion
This study explores preferences for prenatal screening among Australians in both rural and metropolitan areas to assist with the development of an equitable implementation model. While metropolitan preferences were shaped by the accuracy and cost of screening tests, rural preferences were influenced by a broader range of screening features. This study quantifies the impact of intermediate and process outcomes on preferences through willingness to pay estimates, whilst accounting for differences in preferences between metropolitan and rural communities. These estimates highlight the significant value that individuals place on factors such as reduced waiting times and decreased false-positive rates. Incorporating these estimates into economic evaluations is likely to have implications for the cost effectiveness of prenatal screening and to impact policy decisions that rely on such analyses.
Notes
Financial support for this study was provided entirely by a philanthropic PhD scholarship from Bob and Nancy Edwards (to AS) administered through the University of Sydney, Australia. The scholarship agreement ensured the authors’ independence in designing the study, interpreting the data, writing, and publishing the report.
References
ACMG. Noninvasive Prenatal Screening is now Prenatal Cell-Free DNA Screening. 2023. https://www.gimjournal.org/pb-assets/Health%20Advance/journals/gim/Noninvasive-Prenatal-Screening-is-now-Prenatal-Cell-Free-DNA-Screening-Apr2023-T-4-10-1684409134760.pdf.
Norton ME, Jacobsson B, Swamy GK, et al. Cell-free DNA analysis for noninvasive examination of trisomy. N Engl J Med. 2015;372:1589–97. https://doi.org/10.1056/NEJMoa1407349.
Lüthgens K, Grati FR, Sinzel M, et al. Confirmation rate of cell free DNA screening for sex chromosomal abnormalities according to the method of confirmatory testing. Prenat Diagn. 2021;41:1258–63. https://doi.org/10.1002/pd.5814.
Hu Y, Liu W, He G, et al. Clinical utility of expanded NIPT for chromosomal abnormalities and etiology analysis of cytogenetic discrepancies cases. J Assist Reprod Genet. 2022;39:267–79. https://doi.org/10.1007/s10815-021-02351-6.
Hui L, Halliday J. A decade of non-invasive prenatal screening in Australia: National impact on prenatal screening and diagnostic testing. Aust N Z J Obstet Gynaecol. 2023;63:264–7. https://doi.org/10.1111/ajo.13638.
Drysdale K, Scully JL, Kint L, et al. The salience of genomic information to reproductive autonomy: Australian healthcare professionals’ views on a changing prenatal testing landscape. New Genet Soc. 2024. https://doi.org/10.1080/14636778.2024.2332310.
MSAC. Public Summary Socument, Application No.1492. In: Medical Services Adviosry Commitee DoHaAc, (ed.). http://www.msac.gov.au/internet/msac/publishing.nsf/Content/1492-public2019. Accessed Jan 2024.
RANZCOG. Screening and diagnosis of fetal structural anomalies and chromosome conditions (C-Obs 35) https://mcusercontent.com/9fcf2c84a9b0223059e71ea49/files/25cd4b1a-c5bb-ccd4-010b-bd5ecc480a87/C_Obs_35_Fetal_Anomalies_CG_DRAFT_For_Consultation_20240726.pdf (2024).
Buchanan J, Hill M, Vass CM, et al. Factors that impact on women’s decision-making around prenatal genomic tests: an international discrete choice survey. Prenat Diagn. 2022;42:934–46. https://doi.org/10.1002/pd.6159.
Lewis SM, Cullinane FM, Carlin JB, Halliday JL. Women’s and health professionals’ preferences for prenatal testing for Down syndrome in Australia. Aust N Z J Obstet Gynaecol. 2006;46:205–11. https://doi.org/10.1111/j.1479-828X.2006.00567.x.
Cornell P, Armstrong T, Fyfe R, et al. Experiences of non-invasive prenatal screening: a survey study. Aust N Z J Obstet Gynaecol. 2022;62:241–9. https://doi.org/10.1111/ajo.13436.
Bowman-Smart H, Savulescu J, Mand C, et al. ‘Small cost to pay for peace of mind’: women’s experiences with non-invasive prenatal testing. Aust N Z J Obstet Gynaecol. 2019;59:649–55. https://doi.org/10.1111/ajo.12945.
Long S, O’Leary P, Norman R, Dickinson JE. Willingness to pay for expanded non-invasive prenatal screening—an online discrete choice experiment from the perspective of women living in Western Australia. Aust N Z J Obstet Gynaecol. 2024. https://doi.org/10.1111/ajo.13858.
McFadden D. Econometric models for probabilistic choice among products. J Bus (Chicago, Ill). 1980;53:S13–29. https://doi.org/10.1086/296093.
Bridges JFPP, Hauber ABP, Marshall DP, et al. Conjoint analysis applications in health—a checklist: a report of the ISPOR good research practices for conjoint analysis task force. Value in health. 2011;14:403–13. https://doi.org/10.1016/j.jval.2010.11.013.
Marsh K, de Bekker-Grob E, Cook N, et al. How to integrate evidence from patient preference studies into health technology assessment: a critical review and recommendations. Int J Technol Assess Health Care. 2021;37:e75–e75. https://doi.org/10.1017/S0266462321000490.
Di Mattei V, Ferrari F, Perego G, et al. Decision-making factors in prenatal testing: a systematic review. Health Psychol Open. 2021;8:2055102920987455–2055102920987455. https://doi.org/10.1177/2055102920987455.
Laberge A-M, Birko S, Lemoine M-?, et al. Canadian pregnant women’s preferences regarding NIPT for Down syndrome: the information they want, how they want to get it, and with whom they want to discuss it. J Obstet Gynaecol Can. 2019;41:782–91.
Nazaré B, Fonseca A, Gameiro S, et al. Amniocentesis due to advanced maternal age: the role of marital intimacy in couples’ decision-making process. Contemp Fam Ther. 2011;33:128–42.
Salisbury A, Winston H, Johnson A, Pearce A, Howard K, Norris S. Public perspectives around prenatal screening of chromosomal abnormalities: A focus group study comparing metropolitan and rural/regional areas in Australia. Aust N Z J Obstet Gynaecol. https://doi.org/10.1111/ajo.13935.
Liehr T. False-positives and false-negatives in non-invasive prenatal testing (NIPT): what can we learn from a meta-analyses on > 750,000 tests? Mol Cytogenet. 2022;15:36–36. https://doi.org/10.1186/s13039-022-00612-2.
Rose JM, Bliemer MCJ. Constructing efficient stated choice experimental designs. Transp Rev. 2009;29:587–617. https://doi.org/10.1080/01441640902827623.
Orme BK. Getting started with conjoint analysis : strategies for product design and pricing research, 4th edition. ed. Manhattan Beach: Research Publishers LLC, 2020.
Hauber AB, González JM, Groothuis-Oudshoorn CG, et al. Statistical methods for the analysis of discrete choice experiments: a report of the ISPOR conjoint analysis good research practices task force. Value Health. 2016;19:300–15.
Hu W, Sun S, Penn J, Qing P. Dummy and effects coding variables in discrete choice analysis. Am J Agr Econ. 2022;104:1770–88. https://doi.org/10.1111/ajae.12311.
Hulme-Chambers A, Temple-Smith M, Davidson A, et al. Australian women’s experiences of a rural medical termination of pregnancy service: a qualitative study. Sex Reprod Healthc. 2018;15:23–7. https://doi.org/10.1016/j.srhc.2017.11.008.
Doran FM, Hornibrook J. Barriers around access to abortion experienced by rural women in New South Wales, Australia. Rural Remote Health. 2016;16:1–12. https://doi.org/10.22605/RRH3538.
Noonan A, Black KI, Luscombe GM, Tomnay J. “Almost like it was really underground”: a qualitative study of women’s experiences locating services for unintended pregnancy in a rural Australian health system. Sex Reprod Health Matters. 2023;31:2213899–2213899. https://doi.org/10.1080/26410397.2023.2213899.
Hussain R, Tait K. Parental perceptions of information needs and service provision for children with developmental disabilities in rural Australia. Disabil Rehabil. 2015;37:1609–16.
Mullan L, Armstrong K, Job J. Barriers and enablers to structured care delivery in Australian rural primary care. Aust J Rural Health. 2023;31:361–84. https://doi.org/10.1111/ajr.12963.
Hui L, Barclay J, Poulton A, et al. Prenatal diagnosis and socioeconomic status in the non-invasive prenatal testing era: a population-based study. Aust N Z J Obstet Gynaecol. 2018;58:404–10.
Smit AK, Reyes-Marcelino G, Keogh L, et al. ‘There is a lot of good in knowing, but there is also a lot of downs’: public views on ethical considerations in population genomic screening. J Med Ethics. 2021;47:e28–e28.
McKinn S, Javid N, Newson AJ, et al. Clinician views and experiences of non-invasive prenatal genetic screening tests in Australia. 2022.
Beulen L, Grutters JPC, Faas BHW, et al. Women’s and healthcare professionals’ preferences for prenatal testing: a discrete choice experiment. Prenat Diagn. 2015;35:549–57. https://doi.org/10.1002/pd.4571.
Hill M, Johnson JA, Langlois S, et al. Preferences for prenatal tests for Down syndrome: an international comparison of the views of pregnant women and health professionals. Eur J Hum Genet EJHG. 2016;24:968–75. https://doi.org/10.1038/ejhg.2015.249.
Nguyen HM, Baradaran M, Daigle G, et al. Pregnant women’s and policymakers’ preferences for?the expansion of noninvasive prenatal screening: a discrete choice experiment approach study. Health Sci Reports. 2023. https://doi.org/10.1002/hsr2.1516.
Perspectives of pregnant people and clinicians on noninvasive prenatal testing: a systematic review and qualitative meta-synthesis. Toronto, ON, CA: Health Quality Ontario, 2019.
Vanstone M, Yacoub K, Giacomini M, et al. Women’s experiences of publicly funded non-invasive prenatal testing in Ontario, Canada: considerations for health technology policy-making. Qual Health Res. 2015;25:1069–84. https://doi.org/10.1177/1049732315589745.
Sim WS, Yeo SH, Choi C, et al. Preferences for a non-invasive prenatal test as first-line screening for Down Syndrome: a discrete choice experiment. Prenat Diagn. 2022;42:1368–76. https://doi.org/10.1002/pd.6239.
Goranitis I, Best S, Christodoulou J, et al. The personal utility and uptake of genomic sequencing in pediatric and adult conditions: eliciting societal preferences with three discrete choice experiments. Genet Med. 2020;22:1311–9. https://doi.org/10.1038/s41436-020-0809-2.
Drost RM, Paulus AT, Ruwaard D, Evers SM. Valuing inter-sectoral costs and benefits of interventions in the healthcare sector: methods for obtaining unit prices. Expert Rev Pharmacoecon Outcomes Res. 2017;17:77–84.
Nshimyumukiza L, Menon S, Hina H, et al. Cell-free DNA noninvasive prenatal screening for aneuploidy versus conventional screening: a systematic review of economic evaluations. Clin Genet. 2018;94:3–21. https://doi.org/10.1111/cge.13155.
Kuppermann M, Norton ME, Thao K, et al. Preferences regarding contemporary prenatal genetic tests among women desiring testing: implications for optimal testing strategies: Preferences, inclinations, and optimal prenatal testing decision-making. Prenat Diagn. 2016;36:469–75. https://doi.org/10.1002/pd.4808.
Phillips KA, Deverka PA, Marshall DA, et al. Methodological issues in assessing the economic value of next-generation sequencing tests: many challenges and not enough solutions. Value Health. 2018;21:1033–42. https://doi.org/10.1016/j.jval.2018.06.017.
van Overbeeke E, Forrester V, Simoens S, Huys I. Use of patient preferences in health technology assessment: perspectives of Canadian, Belgian and German HTA representatives. Patient-Patient-Center Outcomes Res. 2021;14:119–28.
Mott DJ. Incorporating quantitative patient preference data into healthcare decision making processes: is HTA falling behind? Patient-Patient-Center Outcomes Res. 2018;11:249–52.
Arntsen KA, Blount LG, Dickerson BJ, et al. Patient-centered health technology assessment: a perspective on engagement in health technology assessment by three patient organizations and a health technology assessment body. Int J Technol Assess Health Care. 2022;38: e76.
MSAC. Guidelines for preparing assessments for the Medical Services Advisory Committee. 2021. http://www.msac.gov.au/internet/msac/publishing.nsf/Content/E0D4E4EDDE91EAC8CA2586E0007AFC75/$File/MSAC%20Guidelines-complete-16-FINAL(18May21).pdf.
Acknowledgements
We would like to acknowledge the contribution of the Expert Advisory Group (EAG), whose insights were instrumental in shaping this research. The EAG comprised: Antonia Shand, Karen Mizia, Lisu Hui, Ron Fleischer, Nasrin Jamani Javid and Joanne Scarfe.
Author information
彩票大全软件:Authors and Affiliations
彩票大全软件:Corresponding author
Ethics declarations
彩票大全软件:Credit category
Conceptualisation: A.S., S.N., A.P., K.H.; Data curation: A.S; Formal analysis: A.S.; Funding acquisition: A.S.; Investigation: Not applicable; Methodology: A.S., S.N., A.P., K.H.; Project administration: A.S., S.N., A.P., K.H.; Resources: Not applicable; Software: NA; Supervision: S.N., A.P., K.H.; Validation: NA; Visualisation: NA; Writing-original draft: A.S., S.N., A.P., K.H.; Writing-review & editing: A.S., S.N., A.P., K.H.
彩票大全软件:Funding
Open Access funding enabled and organized by CAUL and its Member Institutions. This study was supported by the Bob and Nancy Edwards Scholarship (SC1217).Footnote 1
彩票大全软件:Competing interests
Authors declare no competing interests
彩票大全软件:Ethics declaration
This research has been approved by the Human Ethics Committee at the University of Sydney [2023/170].
彩票大全软件:Consent to participate
Consent was obtained by all participants prior to starting the survey.
彩票大全软件:Consent for publication
Consent was obtained by all participants prior to starting the survey.
彩票大全软件:Availability of data and material
Data used to support the findings of this study are available from the corresponding author upon reasonable request.
彩票大全软件:Code availability
Available from the corresponding author upon reasonable request.
Supplementary Information
Below is the link to the electronic supplementary material.
彩票大全软件:Supplementary file1 (DOCX 60 KB)
Rights and permissions
Open Access This article is licensed under a Creative Commons Attribution-NonCommercial 4.0 International License, which permits any non-commercial use, sharing, adaptation, distribution and reproduction in any medium or format, as long as you give appropriate credit to the original author(s) and the source, provide a link to the Creative Commons licence, and indicate if changes were made. The images or other third party material in this article are included in the article's Creative Commons licence, unless indicated otherwise in a credit line to the material. If material is not included in the article's Creative Commons licence and your intended use is not permitted by statutory regulation or exceeds the permitted use, you will need to obtain permission directly from the copyright holder. To view a copy of this licence, visit http://creativecommons.org/licenses/by-nc/4.0/.
Reprints and permissions
About this article
彩票大全软件:Cite this article
Salisbury, A., Norris, S., Pearce, A. et al. Australian Preferences for Prenatal Screening: A Discrete Choice Experiment Comparing Metropolitan and Rural/Regional Areas. Appl Health Econ Health Policy 23, 493–506 (2025). https://doi.org/10.1007/s40258-024-00938-5
Accepted:
Published:
Issue Date:
DOI: https://doi.org/10.1007/s40258-024-00938-5